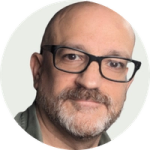
Dr Nick Fine
11 June 2024
AI and user research: How we can (and must) do better
The information wars are real, misinformation is rife, and in comes AI—a genius creation machine at best and a dangerous liar at worst. Sometimes I feel more like a preacher than a scientist.
In user research, we used to spend countless hours manually transcribing interviews before auto-transcription came around—it was game-changing. It’s no wonder researchers are now hoping that AI analysis of those interview transcripts could have a similarly transformative effect on our workload. But I’ve spent far too much time over the past year checking AI output for validity when it was supposed to help alleviate some of that heavy lifting that is characteristic of research work—and trust me, after 15 years of heavy lifting, I’m highly motivated to find an easier way.
So let’s cut to the chase: as of GPT-4o, AI still isn’t helpful for user research work because it consistently and reliably hallucinates and omits. And yet, it is inevitable that its use will become more and more commonplace in every sector, not just my own, and if we’re going to avoid those pitfalls, we all need to learn how to use AI better.
And so I’ve become a preacher—out of necessity rather than ambition—and it has led me to become some kind of reluctant social media influencer. After some time spent sharing my findings and venting my frustration to my LinkedIn network, I was recently invited to speak on the subject at both UX Lisbon and WebExpo in Prague. In sharing my thoughts and some highlights from those events with a wider audience, I hope I can contribute to the ‘better AI’ conversation.
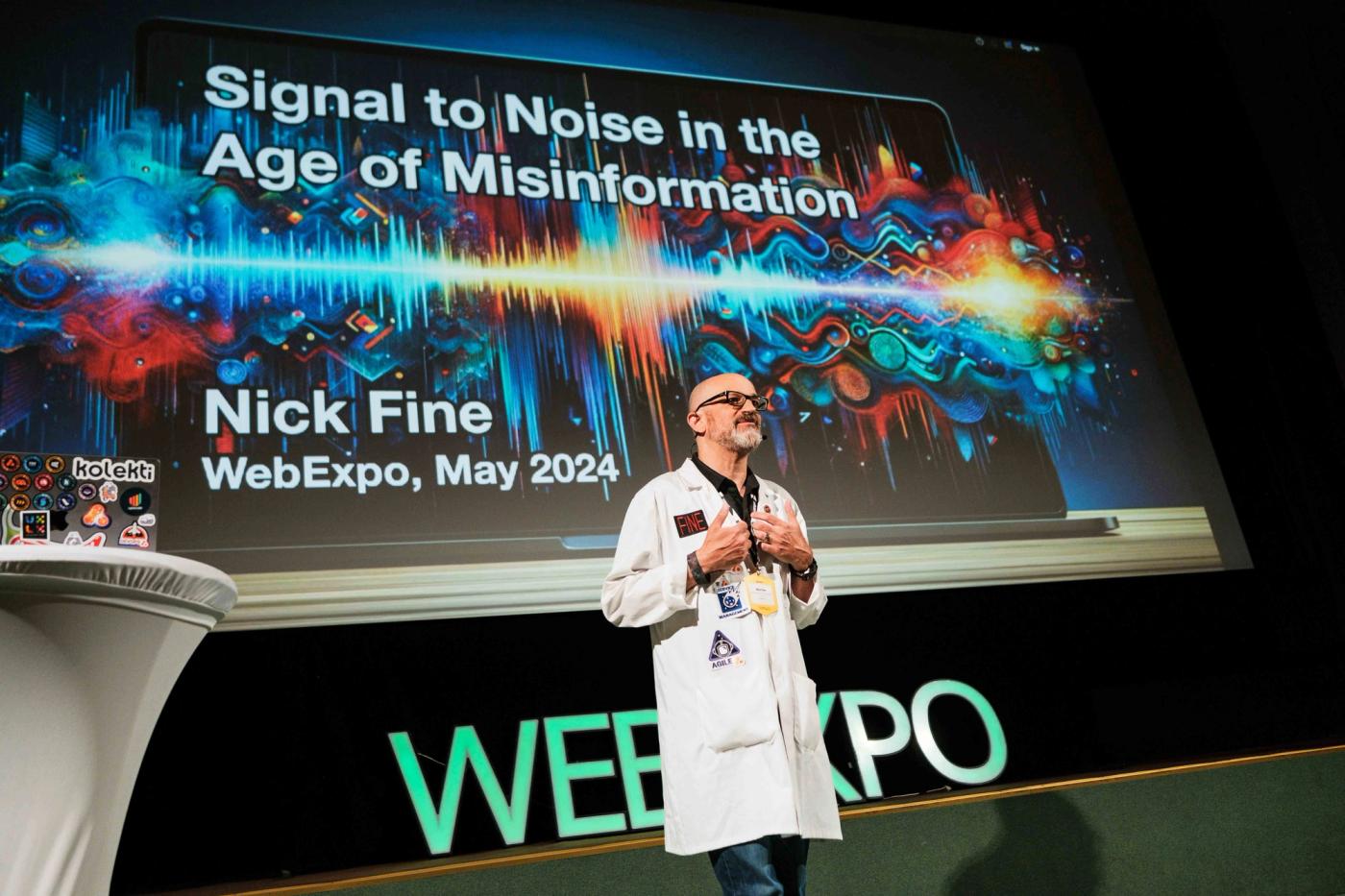
What’s the problem?
I opened my talk in Prague with ‘You don’t eat food that you found on the floor, because you have no idea where it came from—it might make you sick. So why do we put information into our bodies without questioning where it came from?’ The focus of Prague’s talk was on how to spot misinformation, but also the importance of being able to do so for both personal and professional reasons. Effectively, you can’t grow in the right direction if you’re feeding yourself the wrong information. And there’s no doubt that AI’s output often resembles the metaphorical ‘food on the floor’ of that analogy.
Through a user research lens, which is my particular area of both scientific interest and professional pursuit, this is perhaps best illustrated in practice through the course of the half-day workshop I ran in Lisbon.
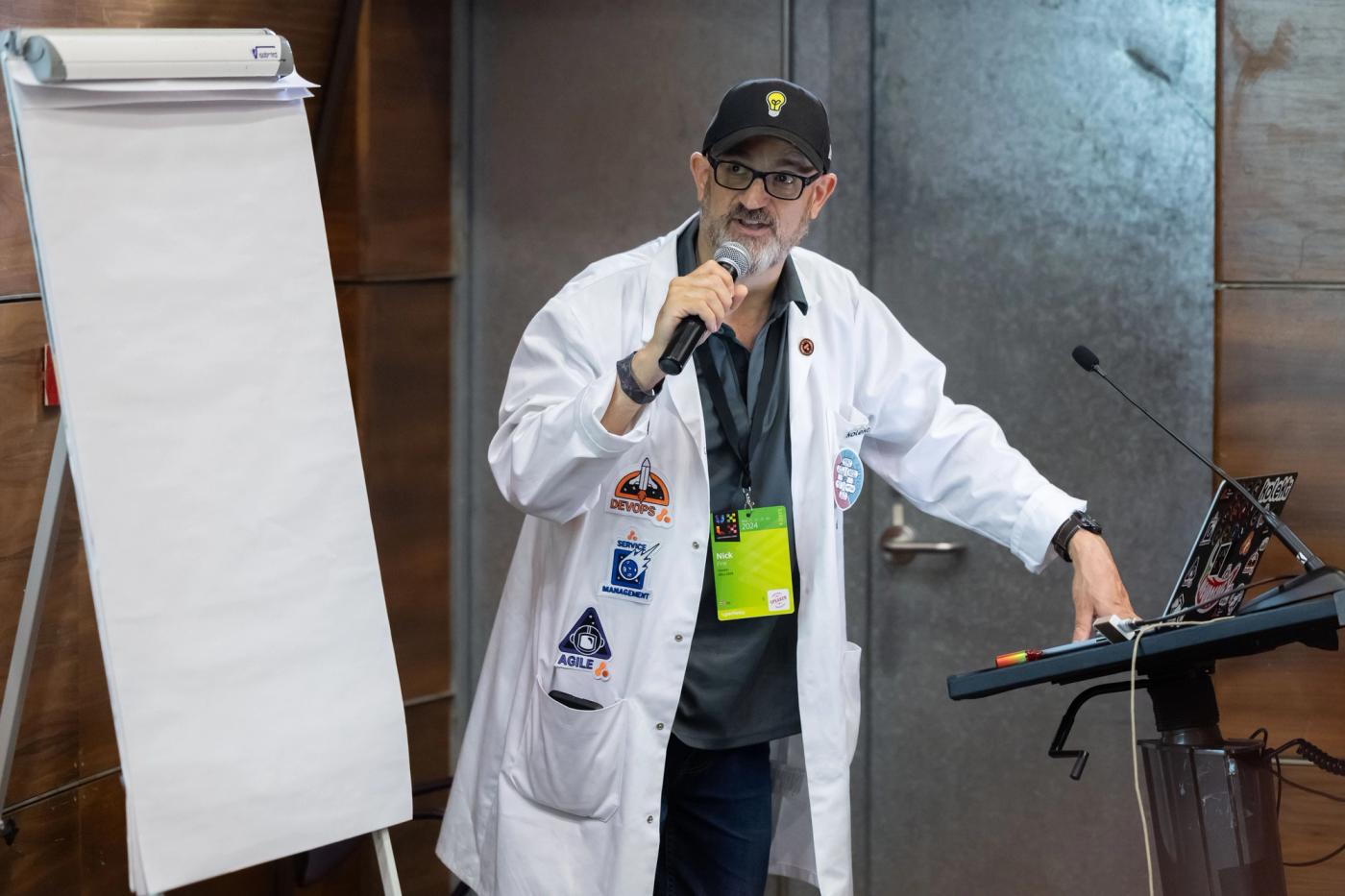
From the outset, I expected the room to be full of delegates hungry to learn the secret of the perfect prompt, but the goal of the workshop was simultaneously to set people up for self-sufficiency and to demonstrate the flaws of that ‘template mindset’. Simply pinching other people’s prompts doesn’t cut it. So, naturally, that’s exactly how we started.
I handed out a section from a genuine transcript of some old private client work of mine (names anonymised, of course) and gave everyone one of my main analysis prompts. After 30 minutes, we compared outputs. At first, people were confident in their findings, but as the discussion continued, the picture started to change. Things weren’t lining up. There were direct contradictions. Two clear examples of omission emerged. The results varied so wildly that all confidence in the AI outputs vanished.
With that, the risk of validity issues presented by AI had been clearly demonstrated, but I would be remiss not to mention another factor for researchers to consider: data protection. There’s a need for far greater awareness about the risks of sending personally identifiable information (PII) and proprietary company information to a public model. My transcript for this exercise was anonymised, but this is something I think people—casual users and professionals alike—with the best intentions still often overlook amid all the excitement and enthusiasm to use these new tools available to us.
The point is that just prompting without thinking is not only inefficient, but dangerous.
Having established these risks and bolstered the motivation to use AI appropriately, I moved on to the role of the user researcher within a team. Rather than simply an ‘order taker’, the user researcher must act as a trusted advisor and a ‘guardian of validity’—you have the responsibility to ensure that your work is scientifically and factually sound—and if you are not fulfilling that role, nobody else is. User research in general, but particularly when exploring emerging technology like AI, requires this level of awareness.
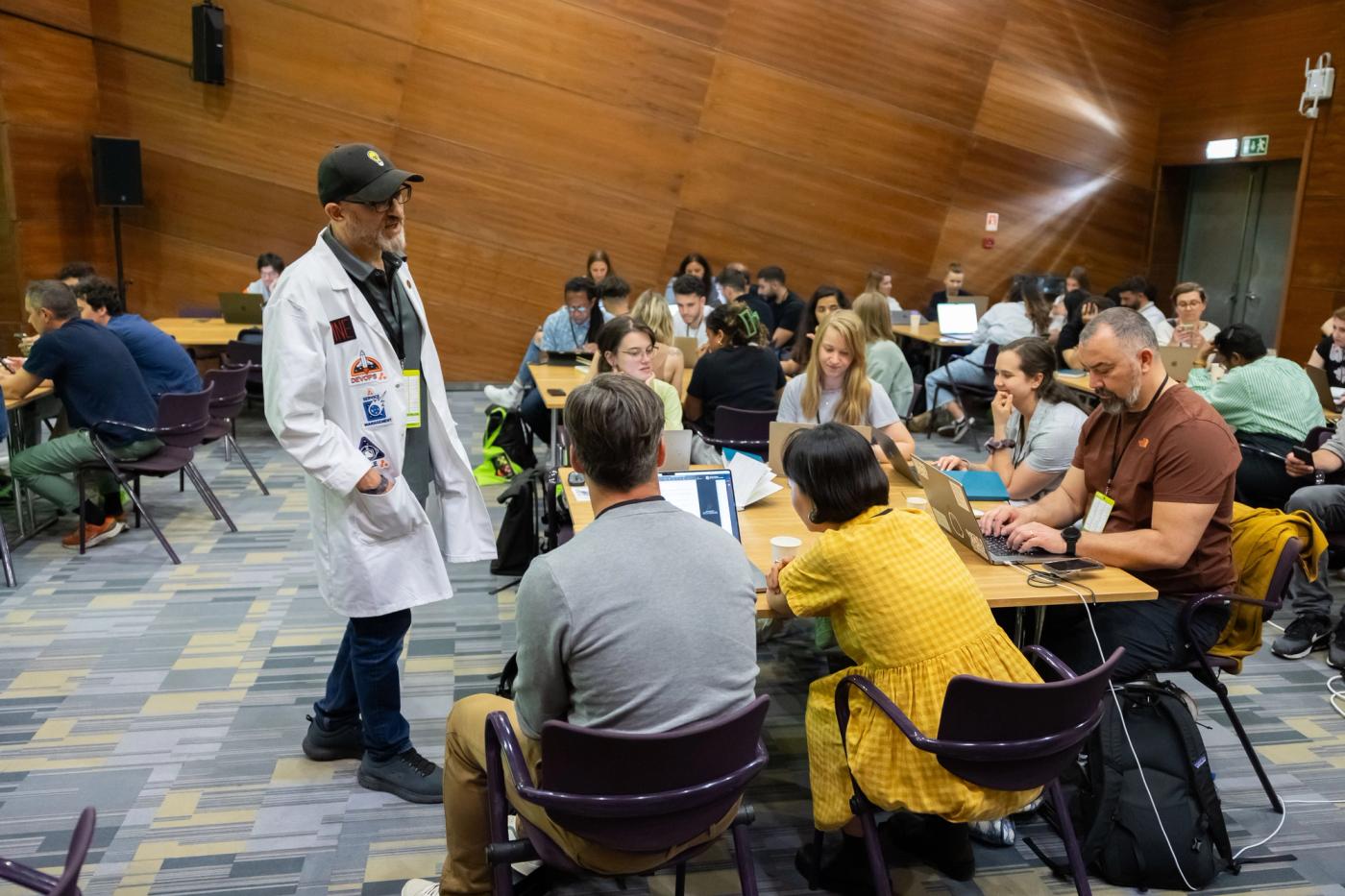
How can we do better?
Enter the concept of AI-Supported User Research: a simple framework for testing and using AI safely and responsibly. I’ve called it AISUR for short. Not the nattiest of acronyms, but it works—I pronounce it ‘eyesore’, if that helps!
AISUR is a straightforward and effective way to introduce good AI behaviours in researchers. It becomes habit very quickly and ensures the protection of both data and researcher, but it relies on the foundation of that validity mindset and effective chunking so that the AI output can be double-checked effectively by human intelligence.
One of the key pillars of AISUR is good planning. Planning research and writing a script for an interview knowing you’re going to use AI to analyse the transcript requires a slightly different approach to traditional, non-AI-supported user research. There’s a skill to writing a script for AI analysis in a way that reduces errors and omissions—because if you give AI half a chance, it’ll happily fill in the blanks with beautiful and highly compelling yet entirely fabricated misinformation.
Another important concept is getting away from the idea that AI usage is ‘one and done’. I teach that AI is usually a crafted experience and requires multiple iterations to get to what you want. Prompting is no different, and the workshop in Lisbon involved the demonstration of two different kinds of secondary prompting: check and probe prompts. By using a combination of these as follow-up prompts, you can check the validity while shaping the output.
I hope that frameworks like AISUR, and on a wider scale, skilled and responsible use of AI generally, will become the norm. I also believe that learning to recognise and challenge low-quality information, or outright misinformation, in all its forms is a vital skill for navigating our modern world. We really need this to be taught more extensively in schools and not as a new concept for professionals.
From these events, I was delighted with the positive feedback from attendees, particularly considering the minefield that is presenting a workshop centred on technology that changes with the wind, and the negative response that can often follow when one takes a decisive stance on such a hot button topic. I’m so grateful to have the opportunity not only to share my own teaching but also to learn so much from other high-level speakers at events like these, and I am enthusiastic at the possibility of exploring more to this end—if I may, Japan, Mexico, or India would all be lovely! In the meantime, regardless of location, I’ll keep fighting the good fight and ‘preaching’ to anyone who will listen.
For more on AISUR or to book Dr Nick Fine for an event, connect on LinkedIn.